- Image annotation techniques like 3D cuboid annotation, segmentation, and polylines hold the key to training computer vision-based models and AI applications in accurate object recognition.
- Professional image annotators use diverse techniques to ensure high-quality annotations that fulfil the specific training needs of AI projects.
- We highlight 13 real-world applications of image annotation across industries detailing use cases and annotation techniques used for each.
Image annotation is essential for AI and machine learning, impacting a broad range of industries from autonomous vehicles to healthcare.
Today, AI systems controlling self-driving cars are trained on billions of accurately labeled images. Healthcare AI is identifying cancer with up to 90% accuracy. And the retail AI market is expected to grow to $23.3 billion by 2027. Precision farming is seeing a yield increase of 20-30%, and the market for video surveillance is projected to reach $74.6 billion by 2025.
This rapid spread of vision-based AI and its critical dependence on image training datasets has brought image annotation under the spotlight. Image annotation is crucial for training AI models accurately, but knowing the what, where, and the how of it can be challenging.
To help AI and ML professionals get a clearer grasp of the current scenario, this article presents 13 industry-wise use cases of image annotation. These provide practical applications to help you improve your model training efforts.
Table of Contents
Use Cases and Techniques of Image Annotation across Industries
Image annotation is widely used across various industries, each leveraging this technology for different applications with proven results. It has transformed how we interact with visual data across these industries, to leverage AI and machine learning applications.
Here are some examples of industries with respective use cases and techniques employed in image annotation tasks:
1. Image annotation for Autonomous Vehicles
Image annotation helps self-driving cars recognize and interpret their surroundings, such as identifying pedestrians, traffic lights, and different types of roads.
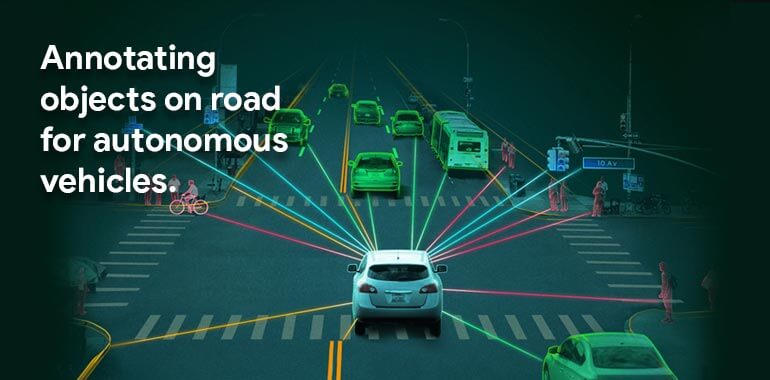
Use Cases
- Object Detection for Pedestrian and Vehicle Recognition: Image annotation helps autonomous vehicles to detect and classify various objects such as pedestrians and vehicles, playing a vital role in navigation and collision avoidance.
- Scene Segmentation for Road Environment Understanding: Through segmenting elements of a road environment, autonomous vehicles gain greater understanding and navigation capabilities in varying traffic conditions.
Techniques
- Bounding Boxes for Vehicles and Pedestrians: This involves outlining vehicles and pedestrians with rectangles to identify and classify them. This basic technique in simple object detection for autonomous vehicles helps the vehicle’s computer vision-based perception model to recognize objects.
- 3D Cuboid Annotation for in-depth Recognition of Objects: 3D cuboid annotation provides depth information, allowing vehicles to sense the distance of objects and avoid collisions.
- Semantic Segmentation for Road Types: This technique labels each image pixel to differentiate between road types, sidewalks, and other elements, helping to understand the scene accurately.
- Polyline Annotation for Lane Detection: Polylines mark lane boundaries, assisting in lane detection and maintaining the vehicle within its lane.
- Polygon Annotation for Irregular Shaped Objects Recognition: It helps in recognizing irregularly shaped objects like road markings, improving the vehicle’s ability to navigate complex environments.
2. Image annotation use case of Healthcare
In the medical field, image annotation is used for labeling and annotating medical images like X-rays, CT scans, and MRIs for identifying anatomical structures and anomalies. This aids in accurate and efficient diagnoses and treatments.
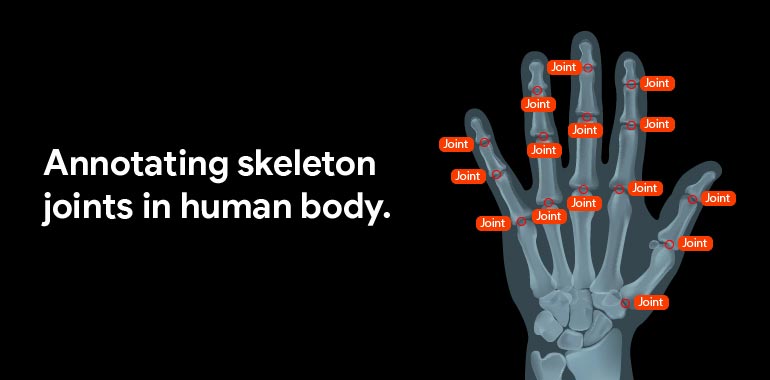
Use Cases
- Identification of Anatomical Structures in Medical Imaging: Image annotation improves medical diagnostics and analysis by helping to recognize and label anatomical structures in X-rays, MRIs, and CT scans.
- Anomaly Detection: Annotating images helps in identifying lesions, tumors, or other abnormalities in medical images, assisting in diagnostics and treatment planning.
Techniques
- Landmark Annotation for Anatomical Structures: Specific anatomical landmarks are marked for their identification and analysis.
- Bounding Boxes for Lesion Detection: This involves drawing rectangular boxes around lesions for efficient detection and measurement.
- Segmentation for Tumor Identification: Segmentation separates tumor regions from the rest of the image for detailed examination and analysis.
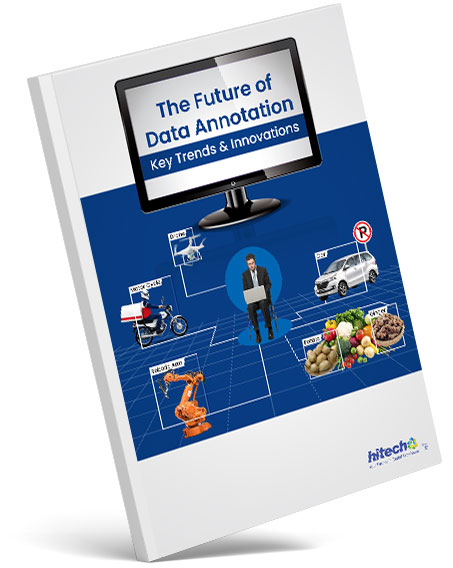
The Future of Data Annotation: Key Trends and Innovations
- Industries globally are impacted by advancements in annotation.
- Smart tools and techniques enhance the performance of ML models.
- Synthetic data and multimodal annotation are trends that will dominate.
- Ethical data annotation practices ensure responsible AI development.
3. Image annotation use case of Retail and E-commerce
The retail industry uses image annotation to identify and categorize products, which improves customer experience through personalized recommendations and inventory management optimization.
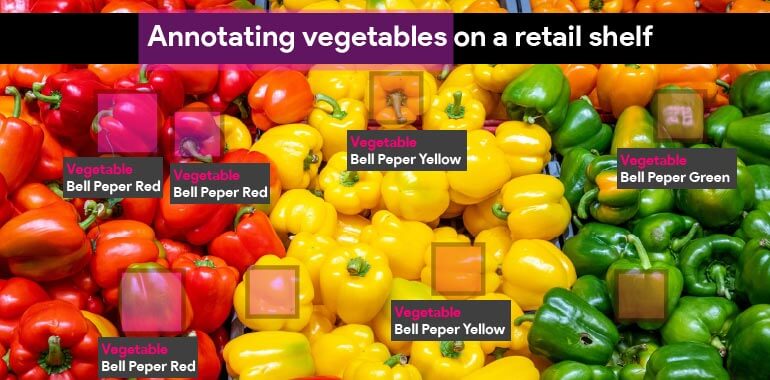
Use Cases
- Product Identification and Categorization: This helps in sorting products and efficient inventory management, ensuring accurate product management.
- Visual Search for Product Discovery: Image-based product searches improves customer experience by allowing them to find products using images. These annotations can include information about the product’s features, such as color, shape, size, texture and other specific attributes.
- Inventory Management: Assists in tracking and organizing products, contributing to efficient inventory control.
Techniques
- Bounding Boxes for Product Identification: This helps to identify and categorize different products, streamlining product management and inventory processes.
- Tagging for Categorization: Products are tagged for easy categorization and searchability, improving the shopping experience.
- Image Classification for Product Sorting: Classifies products into different categories based on visual features, facilitating better product management.
- Enhanced Visual Search: Empowers customers to find products by uploading images, even when they are unable to articulate their requirements verbally.
- Quality Control: Image annotation helps in identifying defective products through visual inspections, improving quality control measures.
- Augmented Reality (AR) Shopping: Annotated images are fundamental in implementing AR in e-commerce, offering customers an immersive shopping experience.
Want high-quality image annotations tailored to your project needs?
Save time and cost with our quick and efficient image annotation workflow.
4. Image annotation use case of Security and Surveillance
Image annotation helps in identifying potential threats and alerting security personnel, thereby helping law enforcement agencies in preventing criminal activities.
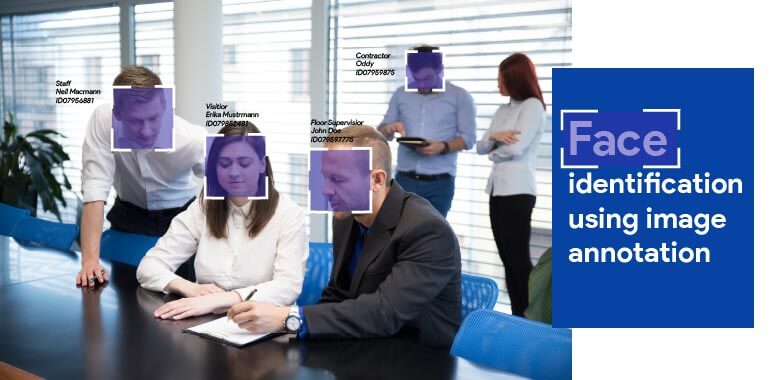
Use Cases
- Threat Detection: Image annotation is used to detect potential security threats in real-time, such as unattended suspicious items, improving area security.
- Suspicious Activity Identification: Helps in recognizing unusual behaviors or individuals in areas of concern, aiding in proactive security measures.
- Real-time Surveillance: Enables real time monitoring of specific areas for security purposes, tracking objects and individuals to ensure safety.
Techniques
- Object Detection Using Bounding Boxes: This technique differentiates people from objects, helping to identify potential threats or items of interest in security footage.
- Facial Recognition Using Landmark Annotation: Employed for identifying individuals using facial features, enhancing security systems’ ability to recognize and track persons of interest.
- Activity Recognition through Sequence Annotation: Analyzing sequences of images to recognize specific activities or behaviors, important for identifying suspicious actions in real-time surveillance.
- Semantic Segmentation for Area Coverage: Divides a whole area into smaller parts in videos, allowing for easier surveillance of different sections and marking restricted areas.
5. Image annotation use case of Agriculture
In agriculture, image annotation increases crop yields and optimizes resource utilization. It assists farmers in monitoring crop growth and identifying pests and diseases.
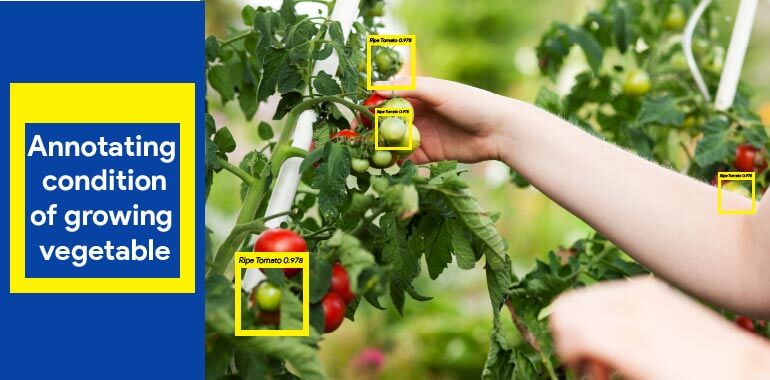
Use Cases
- Crop Health Monitoring: Assessing the health and growth of crops, ensuring optimal agricultural output.
- Pest Detection: Identifying and classifying different types of agricultural pests, facilitating better crop protection.
- Yield Estimation: Estimating the yield of crops based on visual data, aiding in agricultural planning and resource allocation.
Techniques
- Segmentation for Crop Type Identification: Differentiates between crop types, aiding in precise agricultural management.
- Object Detection for Pest Identification: Recognizing and classifying pests, vital for pest control measures.
- Condition Scoring through Image Classification: Assesses the condition of crops based on visual cues, important for monitoring crop health and predicting yields.
- Deep Learning-Based Image Annotation: Utilizes advanced machine learning techniques to analyze large amounts of agricultural image data, providing accurate predictions for complicated and ambiguous situations in agriculture.
6. Image annotation use case of Geospatial Analytics
This involves analyzing spatial data using satellite and aerial imagery, where image annotation helps identify features like buildings, roads, and water bodies, supporting urban planning and environmental studies.
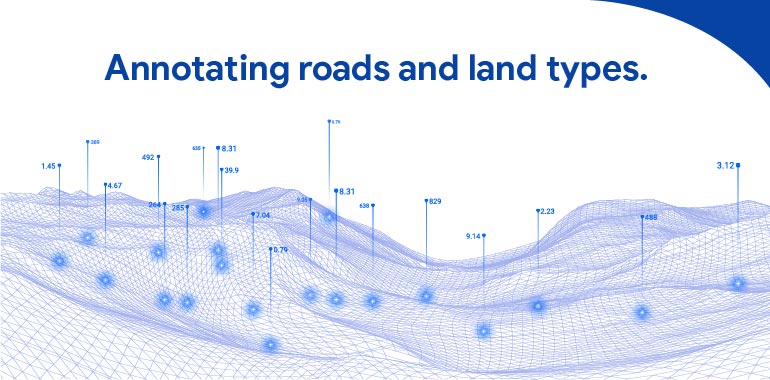
Use Cases
- Land Use Analysis: Understanding how different land areas are being used, essential for environmental and urban planning.
- Environmental Monitoring: Observing changes in the environment, crucial for climate science and disaster management.
- Urban Planning: Promoting sustainable development through assistance in the planning and development of urban areas.
Techniques
- Object Detection for Buildings and Roads: Identifying man-made structures, essential for urban planning and land use analysis.
- Segmentation for Land Cover Classification: Classifying different types of land covers, important for environmental monitoring and analysis.
- Pattern Recognition for Environmental Changes: Detecting changes in the environment over time, crucial for understanding ecological and climatic shifts.
- Image Annotation for Complex Geospatial Imagery: Simplifying and clarifying complex geospatial imagery, which is often captured from aerial, satellite, or remotely-sensed sources, making it actionable for various applications in fields like urban planning and climate science.
7. Image annotation use case of Wildlife Conservation
Image annotation is used to monitor and track endangered species, helping conservationists in habitat conservation and population management.
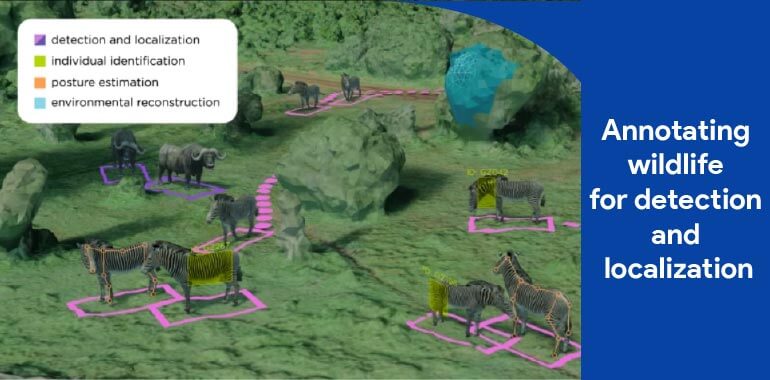
Use Cases
- Tracking Endangered Species: Monitoring movements and populations of endangered animals to better understand and protect them.
- Habitat Monitoring: Observing changes in natural habitats helps in maintaining biodiversity and ecological balance.
- Behavior Analysis: Studying wildlife behaviors for better conservation strategies and understanding of animal habits.
Techniques
- Object Detection for Animal Identification: Using image annotation, different species are identified, aiding in population tracking and species-specific conservation efforts.
- Tracking Annotations for Movement Analysis: Following the movements of animals over time, helping in understanding migratory patterns and habitat usage.
- Segmentation for Habitat Mapping: Mapping out different habitat areas using image segmentation, essential for ecosystem management and protection.
8. Image annotation use case of Social Media
Image annotation is used to tag and identify photographs and videos of people on social media. This helps to personalize their social media feeds and increase user engagement.
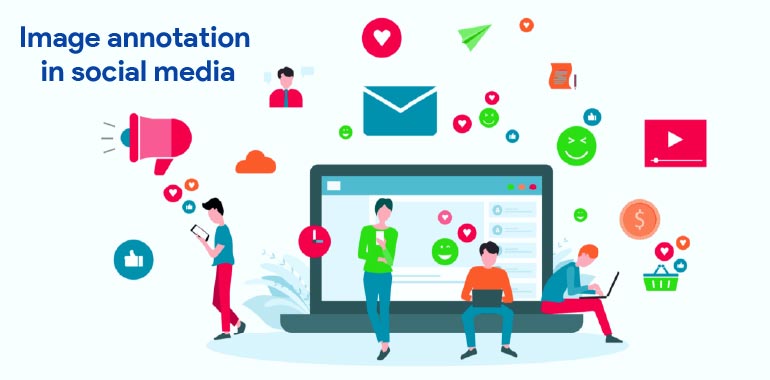
Use Cases
- Content Moderation: Ensuring appropriate content is displayed on platforms.
- User Tagging: Tagging individuals in images for better social interaction and connectivity.
Techniques
- Facial Recognition for Tagging: Identifying individuals in images for accurate and efficient tagging.
- Content Filtering Using Image Classification: Classifying content to ensure appropriateness and adherence to community guidelines.
- Sentiment Analysis through Visual Cues: Analyzing images to understand the sentiment or mood conveyed, enhancing user interaction and content relevance.
9. Image annotation use case of Art and Entertainment
The art and entertainment industry utilizes image annotation for art curation, recommendation systems, and enhancing visual effects in movies and virtual reality experiences.
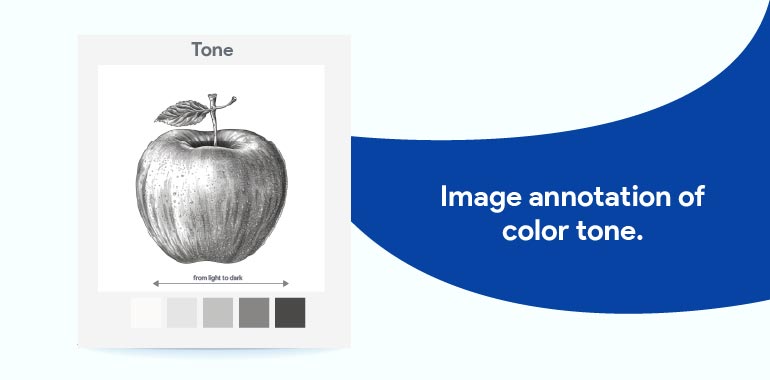
Use Cases
- Art Curation: Image annotation is pivotal in the art and entertainment sector, especially for curators and historians who leverage it to organize and preserve valuable collections by annotating artwork and historical imagery.
- Personalized Content Recommendation: It plays an important role in recommendation systems, suggesting artworks or entertainment content based on users’ preferences. This is achieved through analyzing visual data and aligning them with user preferences.
- Visual Effects in Media: Image annotation enhances visual effects in movies and virtual reality, creating immersive and realistic environments for audiences.
Techniques
- Object Recognition for Artwork Identification: This technique involves identifying and classifying different artworks, aiding in managing and categorizing vast art collections.
- Pattern Recognition for Style Analysis: By analyzing artistic styles and patterns, this method helps in understanding and cataloging various art forms and their historical significance.
- Segmentation for Special Effects: In media, segmentation techniques are used to separate elements in a scene, enhancing visual effects and providing depth and realism to digital imagery.
10. Image annotation use case of Sports Analytics
Image annotation helps in tracking movements and analyzing player actions in sports. This is used in performance analysis and fitness program development.
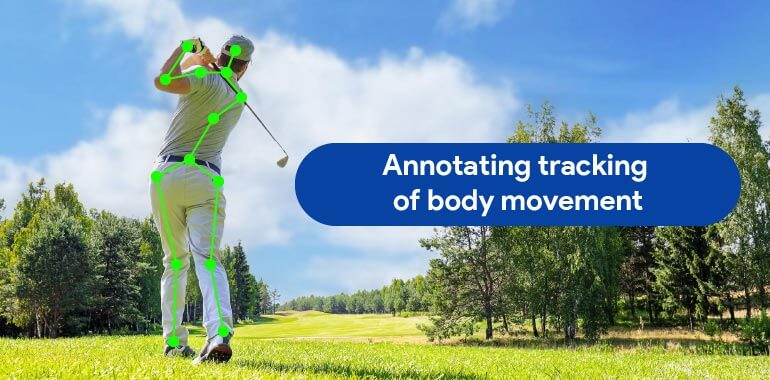
Use Cases
- Performance Analysis: Sports analytics utilizes image annotation to assess athletes’ performance, identifying successful strategies and areas for improvement.
- Player Tracking: Monitoring athletes’ movements and positions during games helps in strategizing and improving team dynamics.
- Injury Prevention: By analyzing players’ movements, potential injuries can be predicted and prevented, ensuring players’ health and longevity in sports.
Techniques
- Pose Estimation for Movement Analysis: This technique involves analyzing athletes’ poses and movements to improve performance and reduce injury risks.
- Player Tracking through Sequence Annotation: Tracking the movement of players over time, this method helps in understanding player dynamics and game strategies.
- Action Recognition for Performance Assessment: Recognizing and assessing specific actions or plays, this technique aids in evaluating players’ performance and decision-making skills.
11. Image annotation use case of Livestock Management
Image annotation assists in livestock health monitoring, animal counting, and identifying specific characteristics, improving efficiency in livestock farming.
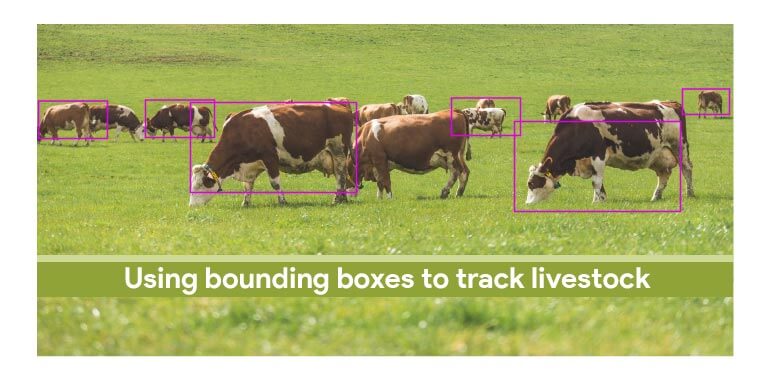
Use Cases
- Health Monitoring: Image annotation is used for monitoring the health of livestock, identifying signs of stress or disease.
- Counting: It aids in keeping track of the number of animals, leading to effective herd management.
- Breed Identification: Identifying and classifying different breeds is another application, crucial for breeding and conservation purposes.
Techniques
- Object Detection for Individual Animal Identification: This method involves identifying individual animals, which is vital for tracking health, growth, and breeding records.
- Pattern Recognition for Health Assessment: By assessing visual patterns, this technique can indicate the health status of animals, helping in early disease detection and treatment.
- Counting Annotations for Herd Management: Counting animals through image annotation helps in effective herd management, ensuring proper resource allocation and monitoring of livestock populations.
12. Image annotation use case of Soil Condition and Geo-sensing
Analysis of soil conditions and geological details, which crucial for agriculture, environmental studies, and natural disaster management is also improved through image annotation.
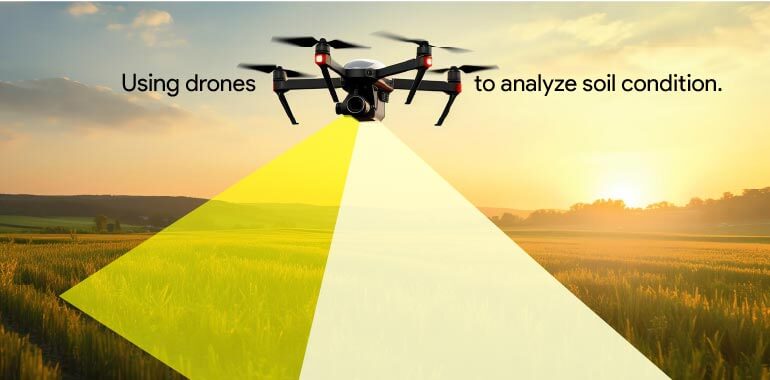
Use Cases
- Soil Quality Assessment: Geo-annotation is used in agriculture for monitoring crop and soil conditions, crucial for assessing soil quality for various agricultural applications.
- Terrain Analysis: This includes analyzing natural topographical features, such as rivers and other water bodies, vital for understanding and managing land resources.
- Environmental Impact Studies: Geo-annotation aids in environmental studies by detecting and predicting different environmental effects on crops and land.
Techniques
- Segmentation for Soil Type Classification: This technique classifies different types of soil, identifying land cover like rivers, lakes, and agricultural lands.
- Pattern Recognition for Terrain Analysis: Utilizing satellite imagery analytics, this method helps in detecting roads, constructions, and analyzing terrain patterns.
- Object Detection for Geological Feature Identification: It’s used for identifying geological features, aiding in urban planning, disaster management, and resource extraction.
13. Image annotation use case of Fashion Analytics
In the fashion industry, image annotation is used for trend analysis, semantic segmentation, and visual search, aiding in categorization and personalized recommendations.
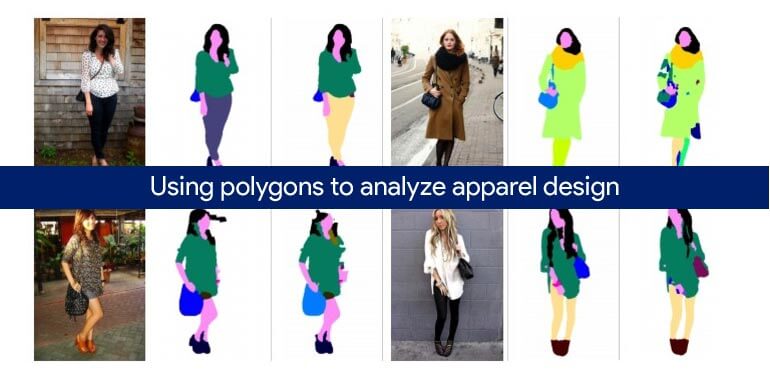
Use Cases
- Trend Analysis: AI-powered virtual fitting rooms and smart visual search applications help in identifying and analyzing fashion trends.
- Style Categorization: Image annotation supports the classification of various fashion styles and trends, aiding in the development of virtual fitting rooms.
- Visual Search: Leveraging image annotation, these technologies enable customers to find fashion items that match their desired look.
Techniques
- Image Tagging for Style Categorization: This involves accurate labeling of clothing items, helping in categorizing different fashion styles.
- Pattern Recognition for Trend Analysis: Pattern recognition algorithms can quickly scan through vast amounts of fashion-related images online, identifying emerging trends. By recognizing patterns such as colors, styles, and materials that are frequently appearing, these algorithms help in pinpointing what’s currently popular.
- Segmentation for Outfit Separation: Semantic segmentation is used to capture smaller or unusual items, such as jewelry, ensuring detailed analysis of fashion items.
Multiple Image Annotation Techniques Used in a Single Use Case
In real-life scenarios, multiple annotation techniques are often combined for a single use case.
Here are some examples of different annotation techniques used with video annotation for the detection of street lanes and traffic:
- Polyline Annotation: This technique helps train computer-based AI tools to recognize street lanes, enabling the machine to see the path, traffic, and diversion. This is useful in the development of high-accuracy autonomous vehicle systems.
- Semantic Segmentation: In street lane and traffic detection, semantic segmentation is used to distinguish between unique elements of a scene, such as vehicles, pedestrians, and different types of lanes. This technique is crucial for autonomous driving systems, as it allows for a detailed understanding of the vehicle’s surroundings.
- Landmark Annotation: In street lane and traffic detection, landmarks are used to identify key features such as traffic signs, road markings, or specific points on the road. This technique can improve the accuracy, precision, and consistency of annotated videos, which is particularly important in autonomous vehicles.
- Instance Segmentation: Instance segmentation is useful in cases, such as in a scene with multiple vehicles, where each vehicle needs to be identified and segmented individually. This is particularly useful in traffic detection, where it’s important to distinguish between individual vehicles.
- Object Detection: In street lane and traffic detection, object detection can be used to identify and track vehicles, pedestrians, and other objects on the road. This technique is crucial for autonomous driving systems as it enables vehicles to recognize objects on the road in real-time.
Each of these techniques has its own strengths and is used in different scenarios depending on the specific requirements of the task. For example, polyline annotation might be more suitable for lane detection, while object detection and instance segmentation might be more suitable for traffic detection. The choice of technique would depend on factors such as the complexity of the scene, the level of detail required, and the computational resources available.
Conclusion
Image annotation bridges the gap between visual data and machine comprehension. From refining object recognition to powering autonomous vehicles, image annotation is revolutionizing entire industries like healthcare, technology, and retail.
As technology advances, image annotation’s significance will only grow, unlocking the full potential of visual data across various domains. It will continue to play an instrumental role in enhancing AI and ML models, reshaping our world and the way we live and work.
Fuel your AI/ML models with high-quality training datasets.
Let us contribute to your computer vision-based models and AI algorithms.