- For B2B data aggregators, data quality is crucial for customer retention, profitability, and analytics-driven businesses.
- Validation, verification, enrichment, and real-time updates directly impact B2B data quality.
- Neglecting these interventions can render B2B data unusable and undermine outcomes of the data aggregation business.
B2B data quality is pivotal to the success of customer retention, profitability, and running analytics-driven businesses. Any lapse in B2B data aggregation process interventions, right from validation and verification to enrichment and near real-time updates, can affect quality and make it unusable for the B2B aggregator.
At the 2019 MIT Citi Conference, Michael Stonebraker, Turing Award winner and Adjunct Prof of Massachusetts Institute of Technology, told industry leaders, “Without clean data, or clean enough data, your data science is worthless.”
This lack of quality data, Stonebraker said, is affecting data science efforts across industries. He held it as the third biggest data science problem of businesses. It comes just after the blunders of not moving data operations to the cloud, and not planning for disruptive AI and ML.
This is just one of the many instances of frustration expressed by top data science leaders about B2B data quality.
At HitechDigital, we have been solving B2B data hygiene issues of a B2B data aggregator for over three decades. And the B2B data quality strategies that we discuss in this article ensure your clients start with high-quality data and that they don’t spend 99% of their time on data wrangling.
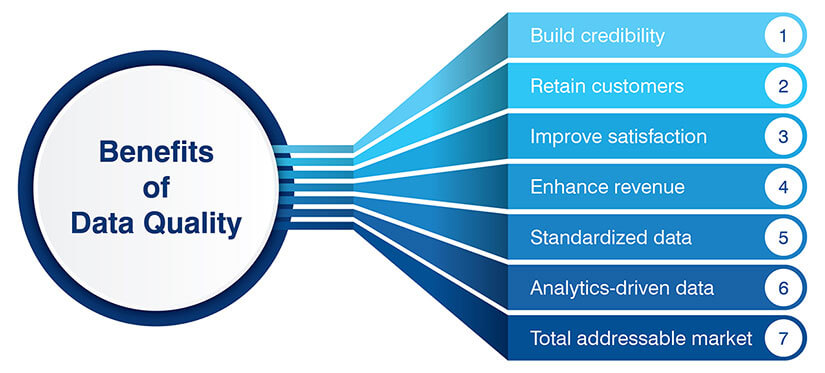
Why do B2B data aggregators face data quality challenges?
Due to the nature of their work, data aggregators have to deal with data from a wide range of sources. The 5 core issues they need to tackle include:
Growing volumes of low-quality unstructured data
Managing the growth of data volumes poses a critical challenge, as does digesting the exponentially growing volumes of unstructured poor-quality data. A report by the International Data Group in 2021 pegged the global growth rate of unstructured data at a shocking 62%. But data aggregators cannot ignore this unstructured data because of the risk of missing out on crucial information relevant to their clients.
Data decay
With time, data loses accuracy. Because it becomes aged, outdated, and thus less authentic. This irrelevant and redundant data can pull down data quality, if it comes from sources that are not updated to current values. As concluded by Gartner in its 2018 report, 3% of global data decays every month. According to DiscoverOrg, it makes individual sales and marketing teams lose an incredible 550 hours every year.
Inauthentic data
Data aggregators work with large databases that stream data at high speeds. Inauthentic data creeps in often during work done at high speed and scale. And sticking to data quality parameters becomes difficult. This continues to be one of the biggest challenges in maintaining B2B data quality.
Incomplete and duplicate data
Any B2B data aggregation work brings in duplicate data, which increases processing loads and errors. B2B records often suffer from missing data. Incomplete and duplicate data increases churn rate, resource consumption, and data maintenance costs. It also creates mail/ delivery errors.
Lack of comprehensive data
Data aggregators work with multiple sets of data. So, for them aggregation of a saleable dataset that meets B2B data quality benchmarks is not possible without the right value additions. Businesses today demand more comprehensive datasets from data selling companies and data enrichment has become a critical concern.
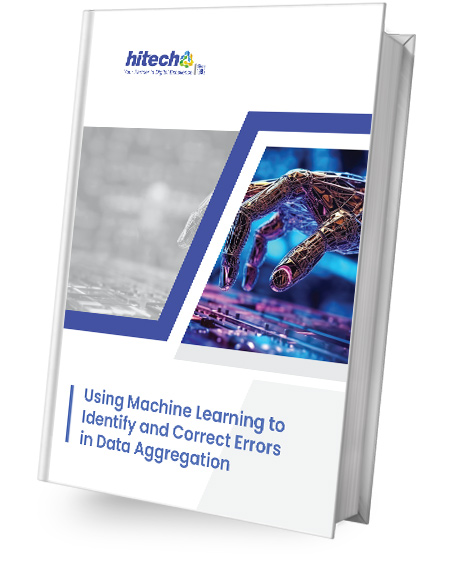
Data aggregation combines information from diverse sources
- Manual methods can’t solely ensure quality of aggregated data.
- Automated error detection improves precision and data quality.
- Combining ML with verification and validation ensures data reliability.
- ML models automate error detection, creating scalable workflows.
Top 5 strategies for data aggregators to ensure optimal B2B data quality
High quality or clean data helps end-clients plan and strategize fruitfully. Thus, working to maintain data quality parameters and strategizing on how to improve data quality have become regular tasks for data aggregators. To make that easy, here are the top 5 strategies to ensure the quality of your B2B database:
1. Enrich data to make it comprehensive
Through data enrichment, we compare and match existing data with third-party and aggregated data. Then create records packed with relevant supplemental information. Data enrichment also includes addressing missing or incomplete information. This makes the information in the databases more comprehensive. Enriched data provides better leads, greater scope for analysis, and higher customer satisfaction.
Perform gap analysis to make data saleable
It is common when dealing with large datasets to find that information required for some specific or targeted usage is missing, or there’s a gap. Through efficient data gap analysis, data aggregators can close these gaps with data enrichment. Then the data matches client objectives and requirements. Gap analysis ensures the efficacy of datasets and increases data saleability.
Match and append missing data
After a thorough gap analysis, clean the required data fields and identify those that need enrichment. Plug in missing values to ensure data integrity. For example, a missing address or a mobile number can reduce the data quality. Empty surnames could misdirect enquiries to the wrong person. Filling in missing information helps enhance B2B data and make the sets accurate.
Capture social graphics and demographic information
Data enrichment spans a variety of demographic, firmographic, and behavioral aspects. We follow usually these two different enrichment approaches. Both techniques work in separate scenarios.
Executive-level enrichment
Includes function, department, and overall executive profiles to help marketers incorporate targets and relevant messages in campaigns.
Account-level enrichment
Monitoring company activities through secondary research, to find demographic, technographic, and job-related data points
Another account-level enrichment strategy includes omnichannel data sourcing
Recently, HitechDigital updated a database of 50 million B2B records for a California-based B2B data aggregator. It empowered them to win over the competition, identify new start-up trends, and find new opportunities.
This approach also reveals information related to revenue, assets, stock information, environmental initiatives, and more. It provides insights into building successful, long-standing relationships.
2. Enhance accuracy to meet data quality parameters
Verification
Data verification involves a process that checks the current data for accuracy, consistency, and relevance. The process is used when data is migrated from outside sources. Most mergers and acquisitions are followed by customer data verification. Only verified data can be merged into the parent company’s system.
Validation
Data validation determines whether a dataset is aligned with the acceptable syntax set for a specific field. This process is often given less attention or priority. This can affect quality. Validating databases aids aggregators reduce risks in selling B2B data.
Validating the database usually involves tasks such as correcting capitalization errors, wrong abbreviations, and misspellings, formats, and conventions. HBR data recently revealed through a survey that bad data causes losses to the tune of a staggering $3 trillion annually. Without validation, data aggregators stand to lose their reputations.
3. Update the database in real time to arrest data decay
Detect data quality issues early
It is essential to address data quality issues right from the time when data sets are initially generated. Every transaction and every act of data migration can have a potential impact on the database.
Detecting data quality issues with the help of verification and validation processes helps a great deal in reducing risks. It also reduces data integrity loss, and the vulnerability of the data sets themselves.
The issues could stem from duplicate data, loads of unstructured data, inauthentic, or incomplete data, erroneous data formats, and other problems. Each of these issues can be weeded out systematically across data aggregation process by data aggregators.
Handle structural errors
Data aggregators need to handle structural errors like unfamiliar naming conventions, typos, or incorrect capitalizations regularly. Many aggregators pay extra attention to weeding out incorrect segmentations, customer profiles, and inaccurate data analysis.
Addressing outliers
Aggregators often encounter information that falls completely outside the normal range. These outliers need to be addressed regularly, as their presence could derail reports, ruin analysis, and cause other harm. Outliers can be from unintentional errors, but aggregators cannot ignore them.
Proactively fix outliers for reliable analytics
The absence of outliers shows proactive interest and action by aggregators. With databases free of outliers, professionals can easily find sizable patterns and trends. Data aggregators should try to spot the origin of specific outliers, whether from individual actions or during data aggregation from a specific source.
This helps them rectify the data collection process and ensure these mistakes do not happen again. Data clean of outliers is of higher utility to data analytics models and algorithms.
4. Ensure comprehensive and all-inclusive updates
Maintain all-round comprehensive information with constant updates
To maintain a comprehensive database of information, it is essential to choose genuine sources. Additionally, these sources need to be varied enough for diversity. Regular updates are crucial in ensuring that the data remains clean and relevant to facts despite changes in the source data.
Eliminate obsolete data sets with authenticated information
To ensure that obsolete data does not rest in the database, aggregators need regular updates. Bad data synchronized across tools is a literal nightmare. Experts recommend identifying and implementing the right sources for the data platform with “whitelisted” segments and accounts. The segments can be synchronized to other tools. This keeps the data fine-tuned with regular updates.
5. Detecting and correcting corrupt / inaccurate records
Standardize data sets
Data standardization ensures relevant standards when aggregating data. Standardization enables the transformation of data based on a consistent format. It could cover capitalization, punctuation, acronyms, characters, and definite values in different fields. The tasks define how the data should be formatted and presented, eliminating extraneous data. And it helps in creating a single view in harmony with the rest of the data sets.
Normalize data sets
Just like data standardization, normalization of data is about changing the numeric values to a common scale. This is done without destroying the context and the relevance. For machine learning algorithms, considering the minimum and maximum values is crucial, especially in relation to presenting data points.
Purge and scrub corrupt data to enable maximum data utilization
Since the lead database needs continuous care, it must be relevant and accurate. B2B data is volatile and can contain corrupted data. These can be in the form of inconsistencies and incomplete data. Ensure your database is populated with the latest valid data, and the rest is archived systematically. Ensure that scrubbing happens and presents tangible benefits.
Merge duplicates to enhance data reputation
Aggregators need to de-duplicate records on a regular basis to prevent them from hogging processing power and wasting resources. Utilize a data deduplication tool to weed out duplicates and ensure that there are single instances of unique records throughout the database.
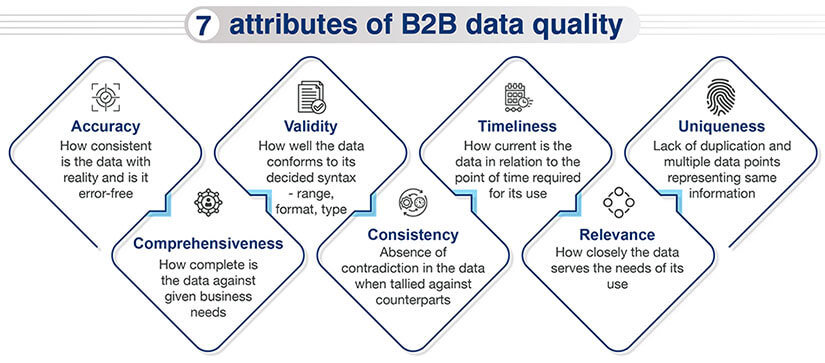
How often is data cleansing necessary for data aggregators to maintain B2B data quality?
Periodic data cleansing is essential for aggregators to keep their existing data from getting dirty and to maintain the quality of the new data they source continuously. The frequency of cleaning varies on the nature of the databases. That said, setting data quality parameters is essential from the start.
The frequency of data being subjected to outside sources plays an important role in assessing the frequency of data quality issue detection.
For example, companies being taken over by a multimillion-dollar company will start finding data quality issues early. Aggregators working with such databases will also have to respond as and when issues are detected. SMEs may require detection and cleansing every 6 months.
A database of Fortune 500 companies would find their data largely intact in terms of its addresses, directors, numbers, and the like. But if some companies are finding dirty data affecting their work, they have start cleansing efforts immediately.
To conclude
B2B Companies confide that the success rate of their sales and marketing campaigns improved considerably due to data quality investments.
Today, the only way forward for B2B data aggregators dealing with huge datasets and big data is to streamline their data aggregation process and inspect all data variables and look for gaps. And organizations need to enrich and enhance their data consistently with regularity.
Thus, for data aggregators, sourcing B2B data properly, cleaning and data quality optimization are a constant part of running operations. You cannot wait around for a customer to appear before you start putting your data into shape. Use these strategies throughout the year to improve B2B data quality and sell data with confidence.