- Implementing robust quality control measures and validation techniques in 3D Scan to BIM projects enhances project management, improves transparency and facilitates remote collaboration.
- Advanced quality control approaches include automation and machine learning techniques for classification, segmentation, and quality control algorithms as well as integration with BIM validation tools.
- Adherence to best practices such as defining SOPs, establishing quality control metrics and thresholds, and documenting and reporting validation results, increases project efficiency.
In the construction industry, accurate assessment of as-built conditions is crucial for successful renovation projects. Scan to BIM (Building Information Modeling) conversion has revolutionized this process by providing surveyors with accurate digital representations of physical structures. However, without proper quality control and validation, the accuracy of BIM models created from scan data can be compromised, leading to significant challenges and errors.
Inaccurate or incomplete point cloud scanned data can result in costly construction delays, with studies estimating an average delay of 14% due to data-related issues. To overcome these challenges, precise Point Cloud Scan to BIM quality and validation techniques are essential. They ensure accurate 3D Scan to BIM conversion and deliver substantial benefits for all stakeholders involved in the design, construction, and management of buildings and infrastructure.
In this article, we explore various quality and validation techniques, as well as their benefits and best practices, for point cloud to BIM. These techniques ensure the creation of accurate and reliable BIM models, ensuring successful renovation, retrofit, and reconstruction projects. Join us as we uncover key insights necessary for achieving accurate and reliable BIM models.
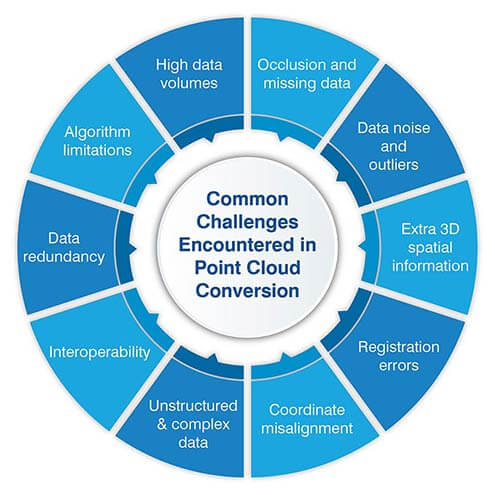
Quality control in 3D Scan to BIM conversion
What is the importance of quality control for accurate BIM models?
Quality control is an integral part of the Point Cloud Scan to BIM conversion process. It is vital to ensure that the captured data is complete, accurate, and reliable. It requires error handling, multiple scans, and accurate geo-referencing. Thus, the Point Cloud to BIM modeling process begins with quality assurance of the point cloud.
In the next phase, we extract information from the point cloud scans for its conversion with software and manual processes. There’s an array of software for the tasks, but it is important to choose tools based on projects. Usually, the choice of tools is left to BIM professionals, who know the software, and have the engineering knowledge needed to assess the project requirements properly.
Finally, the quality of the outputted 3D Revit model needs to be validated. BIM models should represent the physical structure accurately, both geometrically and semantically. This is where the following validation techniques are used.
Pre-conversion quality control techniques
Data pre-processing and cleaning
Before any meaningful work can be done with point cloud scanned data, it often needs to undergo a pre-processing stage. This step involves tasks like file conversion, splitting, or merging datasets, depending on how the data is to be used.
Cleaning is an essential part of pre-processing, where extraneous data, irrelevant for the scope of the project, is removed. This might include points representing objects that were accidentally included in the scan, like passing vehicles or pedestrians. Cleaning reduces the size of the point cloud dataset and helps focus on the relevant data.
Noise reduction and outlier removal
Noise can creep into point cloud data from environmental conditions or errors during scanning, or from limitations of the scanning technology. Noise reduction techniques, such as statistical outlier removal, moving of ‘least’ squares, bilateral filtering, normal estimation, and deep learning-based methods, help to identify and eliminate this noise.
Outliers are data points that deviate significantly from the rest of the data, either because of errors or noise in the data collection process. They can affect the accuracy of the subsequent models if not identified and removed. Various statistical techniques are used for outlier detection and removal. These include statistical outlier removal, spatial depth-pass filter, local density-based methods or learning-based methods, among others.
During-Conversion quality control techniques
Registration and alignment validation
In capturing point cloud data with 3D laser scanners, especially for large scenes or objects, multiple scans are often required. These individual scans need to be combined or “registered” into a single coordinate system.
Registration involves aligning the individual scans relative to each other. It is often done by identifying common features in the overlapping areas of the scans. This process should be validated to ensure that the individual scans align correctly and that there are no distortions or gaps in the combined point cloud.
Checking data completeness and density
Quality control during conversion also involves checking for data completeness and density. Data completeness refers to ensuring that all necessary areas of the object or scene have been scanned and included in the point cloud data. Missing data can lead to inaccuracies in the subsequent models.
Data density refers to the number of data points within a volume. Higher data density can yield more detailed models, but it also increases the data volume and the processing time. Ensuring appropriate data density for the project requirements is a key aspect of quality control.
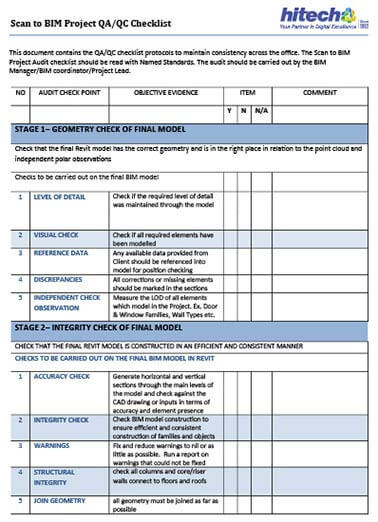
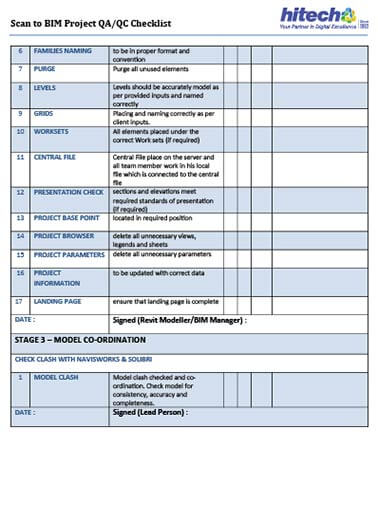
Validation techniques in 3D Scan to BIM conversion
Checking geometric accuracy
Comparison with ground truth measurements
To validate the geometric accuracy of the BIM model, we compare them with “ground truth” measurements that are known to be accurate. This involves comparing the model’s dimensions, locations, or orientations of features with those found in the actual physical object or scene.
This comparison can help identify any systematic errors or biases in the point cloud scanned data or the conversion process. It can then be corrected to improve the model’s accuracy.
Statistical analysis and deviation assessment
Another approach to checking geometric accuracy involves statistical analysis. This is useful when dealing with large datasets where individual comparisons with ground truth measurements are not workable.
A common statistical technique is deviation assessment, where the differences (or deviations) between the point cloud data and the BIM model are calculated and analyzed. Tools can color-code the deviations, making it easier to identify areas where the model significantly deviates from the point cloud data.
Semantic validation and classification
Object recognition and classification
Semantic validation refers to ensuring that the BIM model accurately represents the non-geometric attributes of the object or scene, such as the materials, functional characteristics, and so on.
A key aspect of semantic validation is object recognition and classification. Using machine learning algorithms and other computational methods, the points in a point cloud can be grouped into distinct objects. These objects can be classified into categories like walls, doors, windows, and so forth.
Eliminating false positives and negatives
As with any automated classification process, object recognition can cause errors. False positives occur when the system incorrectly identifies a set of points as an object of a particular type. False negatives occur when the system cannot identify an object.
Validation should involve checking for these errors and correcting them. This may involve tuning the classification algorithm or manually reviewing and correcting classification result.
Model validation and conformance
Comparing the BIM Model with the Point Cloud Scanned Data
One of the most straightforward ways to validate the BIM model is to compare it with the original point cloud data. This comparison can help identify areas where the model does not accurately represent the data.
This process involves inspecting for discrepancies by overlaying of the BIM model onto our point cloud data in a 3D visualization environment. Differences can highlight areas where the model needs to be adjusted to better match the point cloud data.
Identifying Discrepancies and Inconsistencies
Even when the BIM model closely matches the point cloud data, there might still be discrepancies or inconsistencies that need to be addressed.
These can include geometric discrepancies, like gaps or overlaps between features, or semantic inconsistencies, like a door being classified as a window. Identifying and correcting these issues is an important part of the validation process.
Validation techniques ensure geometric accuracy, semantic information, and model conformance with the original point cloud, improving BIM model quality.
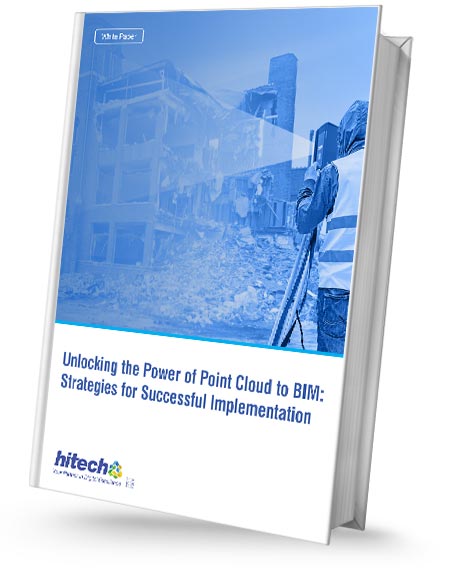
Learn how Point cloud to BIM can streamline your building processes.
- Establish an accurate geospatial context for point cloud data.
- Simplify and optimize mesh representations of point clouds.
- Implement rigorous quality control procedures throughout the process.
- Adopt an iterative approach to modeling based on ongoing analysis.
The benefits of implementing robust quality control measures in Scan to BIM conversion
Implementing quality control and validation techniques in Scan to BIM projects offers several benefits:
- Enhanced Project Management: Point cloud scan to BIM modeling provides a precise method for building highly realistic and interactive 3D models, allowing for better tracking of project progress and decision-making.
- Improved Transparency, Communication, and Collaboration: BIM models generated from point cloud data facilitate better communication and collaboration among project stakeholders, leading to more reliable and efficient project execution.
- Quality Assurance: The accuracy of point cloud scanned data ensures the BIM model reflects the actual construction conditions, providing a higher level of quality assurance.
- Cost Reduction: Implementing a Scan to BIM transformation can help reduce overall surveying and construction costs by providing accurate and detailed information for project planning and execution.
- Remote Collaboration: Point cloud to BIM modeling services enable remote collaboration during both project design and construction, allowing teams to work together effectively even when not physically present at the project site.
- Clash Coordination and Accurate Modeling: 3D Scan to BIM modeling ensures accurate modeling, clash coordination, and generation of precise quantities and bills of materials, which are essential for efficient project execution.
- Drift Invariant Metric Quality Control: By efficiently matching BIM and point cloud data, drift invariant metric evaluation can be performed, providing an intuitive visualization of the construction site’s quality control.
- Facility Maintenance and Operation: An accurate BIM model, reflecting not only the design but also the actual construction, provides invaluable information for future maintenance and operation of the facility.
Advanced quality control and validation approaches
Automation and machine learning techniques
Machine learning-based classification and segmentation
Traditionally, classifying and segmenting point cloud scanned data to distinguish various objects and features has been a manual and time-consuming process. However, recent advances in machine learning have provided automated approaches to these tasks.
Machine learning algorithms can learn patterns and characteristics from labeled training data, and then use these learned patterns to classify or segment new, unlabeled data. In point cloud data, this could involve distinguishing between different types of objects (like walls, windows, and doors) or identifying individual objects within the data.
Automating these tasks not only significantly reduces the time required but also improves consistency and repeatability, as the machine learning models can apply the same rules consistently across the entire dataset.
Automated quality control algorithms
Alongside classification and segmentation, machine learning and other computational methods can also automate various aspects of quality control. For instance, algorithms can be designed to detect noise or outliers in the data automatically. They can also be used to assess the completeness or density of the data, or validate the alignment and registration of multiple scans.
Automating these tasks can speed up the quality control process and improve its consistency. However, it’s important to note that automated quality control algorithms are not infallible. They should typically be used with manual quality checks.
Integration with BIM validation tools
Using BIM software for validation
There are several software tools available for creating, editing, and validating BIM models. These tools can import the BIM model and provide a variety of validation functions.
For instance, BIM validation tools can provide 3D visualization capabilities that allow users to navigate the model in a virtual environment. This makes it easier to identify errors or discrepancies. They can also help check the geometric accuracy of the model, validate the semantic attributes of the objects in the model, or compare the model with the original point cloud data or other reference data.
Clash detection and conflict resolution
A unique feature of many BIM validation tools is the ability to perform clash detection. In BIM, a “clash” refers to a conflict or interference between different elements of the model, such as two structural elements occupying the same space. Clashes and conflicts can also be found between laser scan data and the BIM model, or supplementary information like available plans and construction drawings and the BIM model. All these discrepancies need to be resolved.
Clash detection algorithms can automatically identify these conflicts, which can then be resolved by adjusting the model. This capability is valuable in complex projects involving multiple elements and components.
Best practices for quality control and validation of 3D Scan to BIM modeling
Defining standard operating procedures (SOPs)
Standard Operating Procedures (SOPs) are detailed, written instructions to achieve uniformity of performing a specific function. In Scan to BIM conversion, SOPs can help ensure consistency and accuracy throughout the process.
SOPs should cover all steps of the conversion process, from data collection to pre-processing, conversion, and validation. They should detail the procedures for handling and processing data, performing quality checks, resolving errors or discrepancies, and validating the final models.
Having well-defined SOPs can help reduce errors, increase efficiency, and ensure that all team members understand their roles and responsibilities. They also provide a framework for training new team members and can be used as a reference in case of disputes or disagreements.
Establishing quality control metrics and thresholds
Quality control metrics are quantifiable measures used to assess the quality of the 3D Revit models. These could include measures of geometric accuracy, semantic accuracy, data completeness, data density, and so on. It is at this time that the required level of detail (LOD) of the model is also agreed upon.
Quality control thresholds are pre-defined acceptable limits or ranges for these metrics. For instance, a threshold might be set for the maximum acceptable deviation from the ground truth measurements, or the minimum acceptable data density.
Establishing these metrics and thresholds can provide clear, objective criteria for assessing the quality of the BIM models. They can also help identify potential issues early, allowing for corrective actions to be taken before the issues become more serious.
Documenting and reporting validation results
Documenting and reporting the validation results is a crucial part of the quality control process. This should involve maintaining detailed records of the validation checks performed, the results, any issues identified, and the actions taken to resolve these issues.
This documentation provides a record of the quality control process, which can be useful for auditing purposes, for future reference, or for learning and improving the process. It also provides transparency and accountability, as it shows that the validation checks were performed and that the models meet the established quality criteria.
Reports should be clear and concise, and they should be communicated to all relevant stakeholders, including team members, project managers, clients, and so forth.
Case Studies: Successful implementation of quality control and validation techniques
Case study 1: Coffee Shop Chain Outlet Renovation in the US
A project for creating As-built models with 10mm tolerance from point cloud data to drive renovation efficiencies for outlets of a coffee shop chain in the US. Check out this real-life project.
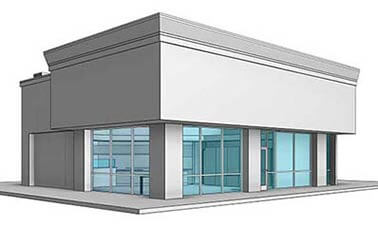
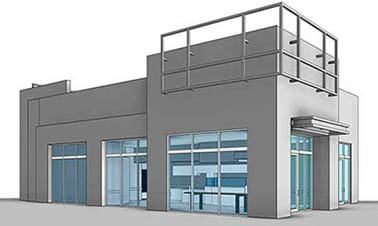
Case study 2: Underground Railway Tunnel in UK
Creating detail-rich 3D Point cloud to BIM model converted from point cloud scans to help efficient renovation of an underground railway tunnel in the UK. Check out this real-life project.
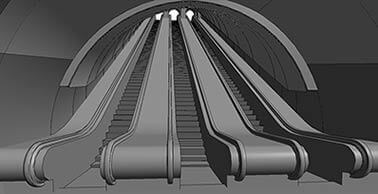
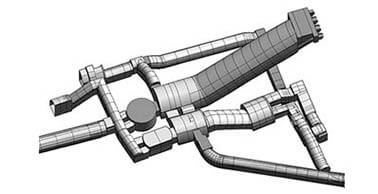
Future trends and challenges – The role of automated tools and algorithms in quality control and validation
Advancements in Scan and BIM technologies
The fields of point cloud scanned data and BIM are rapidly evolving, with new technologies and techniques being developed on a regular basis.
For example, advancements in scanning technologies are leading to more detailed and accurate point cloud data. This includes improvements in LiDAR (Light Detection and Ranging) sensors, photogrammetry techniques, and other remote sensing technologies.
On the BIM side, we are seeing more sophisticated software tools that can handle larger and more complex datasets, and that offer more advanced features for modeling, analysis, and visualization. There are also trends toward more interoperability between different BIM software tools, which can make it easier to work with and share BIM models.
Integrating artificial intelligence and machine learning
Artificial intelligence (AI) and machine learning are increasingly being integrated into the point cloud to BIM conversion processes. As already mentioned, machine learning techniques can automate tasks like object recognition and classification, quality control checks, and even the creation of 3D Revit BIM models from point cloud scanned data.
As these technologies continue to evolve and mature, we can expect them to play an even bigger role in the future. For instance, we might see more advanced AI algorithms that can handle more complex tasks, or that can learn and adapt to new situations more effectively.
However, integrating AI and machine learning also comes with challenges, such as the need for large amounts of labeled training data, the risk of over-fitting or under fitting the models, and the difficulties in understanding and explaining the decisions made by complex AI models.
Overcoming limitations and addressing emerging challenges
While Scan to BIM conversion has come a long way, there are still limitations and challenges that need to be overcome.
One major limitation is the processing time and computational resources required to handle large point cloud datasets and to perform complex tasks like creating BIM 3D models. As the amount of data being collected continues to grow, this challenge is likely to become even more significant.
Another challenge is ensuring the quality and accuracy of the BIM models, especially when dealing with complex or unusual objects or scenes. As already discussed, quality control and validation are crucial parts of the conversion process, but they can be time-consuming and error-prone.
Emerging challenges could include dealing with new types of data or data sources, adapting to changes in standards or regulations, or addressing the needs of new applications or industries.
Despite these challenges, the future of Scan to BIM conversion looks promising. With ongoing advancements in technology, techniques, and best practices, we can expect to see more accurate, efficient, and flexible conversion processes in the future.
Ensuring accurate and reliable 3D Scan to BIM conversion through effective quality control techniques
Quality control and validation are crucial in the Point Cloud to BIM conversion process. They help ensure the accuracy, reliability, and usability of the BIM models, ultimately leading to better project outcomes. Quality control and validation also contribute to more efficient workflows, improved decision-making, and increased confidence in the models, thereby enhancing the value of BIM for design, construction, and facility management.
As this technology continues to advance, it is expected that these processes will become even more refined, leading to more accurate and efficient BIM models. Integration with AI and ML would result in reduced project costs, shorter project timelines, and improved building performance.
Overall, the future of Scan to BIM conversion looks promising, with significant potential to drive innovation and improve outcomes in the architecture, engineering, and construction industry.
Need 3D Scan to Revit models for your renovation project?
Get in touch with our BIM experts NOW →